Applied and Computational Mathematics Seminars
Nov 08, 2024 02:00 PM
328 Parker Hall
Speaker: Alexander Watson (University of Minnesota Twin Cities)
Title: Multiple-scales perspective on moiré materials
Abstract: In recent years, experiments have shown that twisted bilayer graphene and other so-called "moiré materials" realize a variety of important strongly-correlated electronic phases, such as superconductivity and fractional quantum anomalous Hall states. I will present a rigorous multiple-scales analysis justifying the (single-particle) Bistritzer-MacDonald PDE model, which played a critical role in the prediction of these phases in twisted bilayer graphene. The significance of this model is that it has moiré-periodic coefficients even when the underlying material is aperiodic at the atomic scale. This allows moiré materials to be studied via Floquet-Bloch band theory, a variant of the Fourier transform. I will then discuss generalizations of this model and other mathematical questions related to moiré materials.
Oct 29, 2024 02:00 PM
228 Parker Hall
Speaker: Dr. Jennifer Deang (Lockheed Martin; affiliated faculty member of DMS)
Title: On the Mathematical Perspective of the Missile Defense System
Abstract: We first provide an overview of the systems, weapons, and technology needed for detection, tracking, interception, and destruction of attacking missiles. Then we will outline the current research areas sought by the MDA to advance and solve complex technological problems, ultimately contributing to a more robust Missile Defense System (MDS).
DMS Applied and Computational Mathematics Seminar
Oct 25, 2024 01:00 PM
328 Parker Hall
Speaker: Ziad Musslimani (Florida State University)
Title: Space-time nonlocal integrable systems
Abstract: In this talk I will review past and recent results pertaining to the emerging topic of integrable space-time nonlocal integrable nonlinear evolution equations. In particular, we will discuss blow-up in finite time for solitons and the physical derivations of many integrable nonlocal systems.
DMS Applied and Computational Mathematics Seminar
Oct 04, 2024 11:00 AM
328 Parker Hall
Speaker: Dr. Lili Ju (University of South Carolina)
Title: Level Set Learning with Pseudo-Reversible Neural Networks for Nonlinear Dimension Reduction in Function Approximation
Abstract: Inspired by the Nonlinear Level set Learning (NLL) method that uses the reversible residual network (RevNet), we propose a new method of Dimension Reduction via Learning Level Sets (DRiLLS) for function approximation. Our method contains two major components: one is the pseudo-reversible neural network (PRNN) module that effectively transforms high-dimensional input variables to low-dimensional active variables, and the other is the synthesized regression module for approximating function values based on the transformed data in the low-dimensional space. The PRNN not only relaxes the invertibility constraint of the nonlinear transformation present in the NLL method due to the use of RevNet, but also adaptively weights the influence of each sample and controls the sensitivity of the function to the learned active variables. The synthesized regression uses Euclidean distance in the input space to select neighboring samples, whose projections on the space of active variables are used to perform local least-squares polynomial fitting. This helps to resolve numerical oscillation issues present in traditional local and global regressions. Extensive experimental results demonstrate that our DRiLLS method outperforms both the NLL and Active Subspace methods, especially when the target function possesses critical points in the interior of its input domain.
DMS Applied and Computational Mathematics Seminar
Sep 27, 2024 01:00 PM
328 Parker Hall
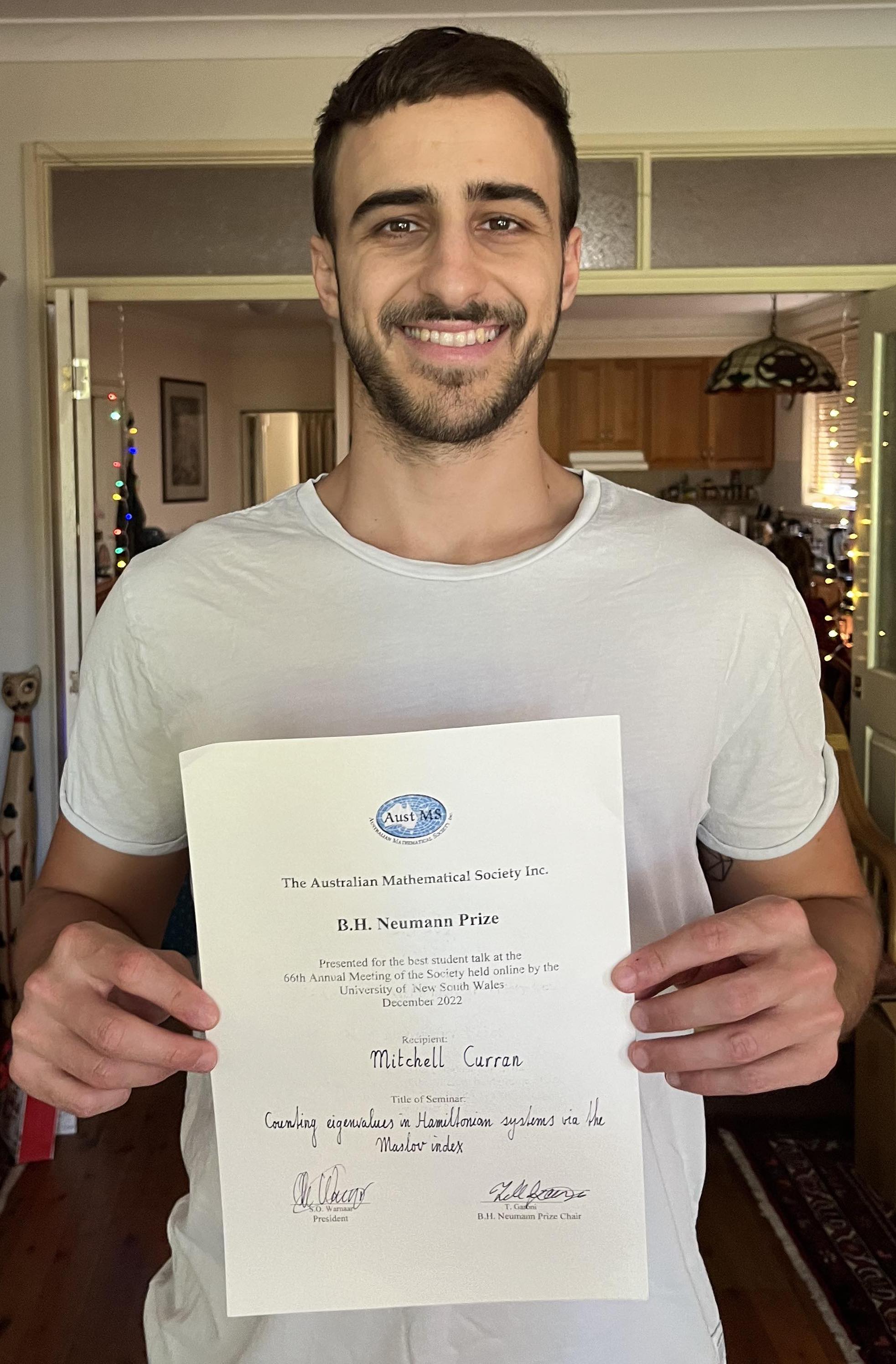
DMS Applied and Computational Mathematics Seminar
Sep 20, 2024 01:00 PM
328 Parker Hall
Speaker: Cao-Kha Doan (Auburn)
Title: Dynamically regularized Lagrange multiplier schemes with energy dissipation for the incompressible Navier-Stokes equations.
Abstract: In this work, we present efficient numerical schemes based on the Lagrange multiplier approach for the Navier-Stokes equations. By introducing a dynamic equation (involving the kinetic energy, the Lagrange multiplier, and a regularization parameter), we reformulate the original equations into an equivalent system that incorporates the energy evolution process. First- and second-order dynamically regularized Lagrange multiplier (DRLM) schemes are derived based on the backward differentiation formulas and shown to be unconditionally energy stable with respect to the original variables. The proposed schemes require only the solutions of two linear Stokes systems and a scalar quadratic equation at each time step. Moreover, with the introduction of the regularization parameter, the Lagrange multiplier can be uniquely determined from the quadratic equation, even with large time step sizes, without affecting the accuracy and stability of the numerical solutions. Various numerical experiments including the Taylor-Green vortex problem, lid-driven cavity flow, and Kelvin-Helmholtz instability are carried out to demonstrate the performance of the DRLM schemes. Extension of the DRLM method to the Cahn-Hilliard-Navier-Stokes system will also be discussed.
DMS Applied and Computational Mathematics Seminar
Sep 06, 2024 01:00 PM
328 Parker Hall
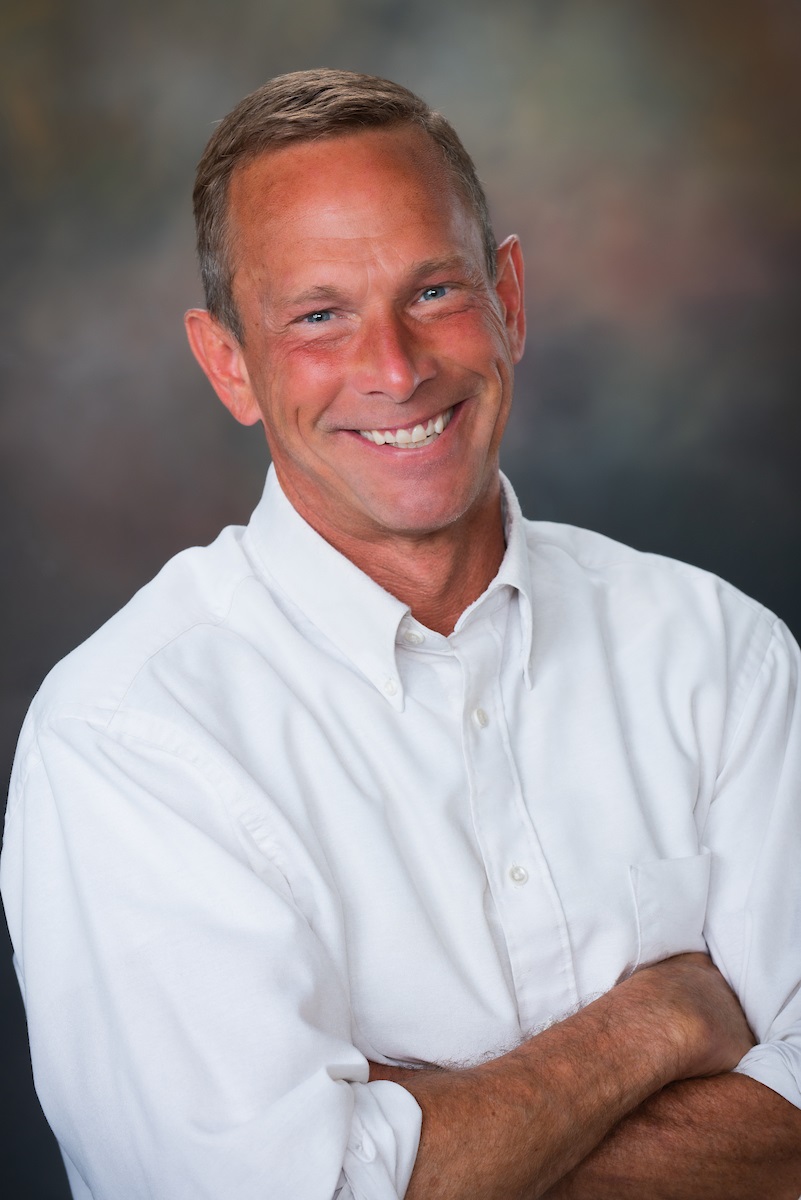
DMS Applied and Computational Mathematics Seminar
Apr 26, 2024 02:00 PM
328 Parker Hall
Speaker: Yuanzhe Xi (Emory)
Title: Acceleration methods for scientific and data science applications
Abstract: There has been a surge of interest in recent years in general-purpose `acceleration' methods that take a sequence of vectors converging to the limit of a fixed point iteration, and produce from it a faster converging sequence. A prototype of these methods that attracted much attention recently is the Anderson Acceleration (AA) procedure. In this talk, we will discuss a new class of nonlinear acceleration algorithms based on extending conjugate residual-type procedures from linear to nonlinear equations. The main algorithm has strong similarities with Anderson acceleration as well as with inexact Newton methods- depending on which variant is implemented. We will demonstrate the efficiency of the proposed method on a variety of problems from simulation experiments to deep learning applications.
This is joint work with Yousef Saad, Huan He, Ziyuan Tang, and Shifan Zhao.
DMS Applied and Computational Mathematics Seminar
Apr 19, 2024 02:00 PM
328 Parker Hall
Speaker: Akil Narayan (University of Utah)
Title: Model selection, combination, and management: Sowing with exploration and reaping with exploitation
Abstract: Modern simulation-based scientific models are complex and multi-faceted, involving computationally demanding physics-based modeling and discretization, reliable and robust data assimilation, and an accurate accounting for uncertainty in the face of unknown model parameter values and/or genuine stochasticity. To meet such demands, many simulations of real-world systems often involve combinations of homogenized, microscale, or reduced order model components that target accuracy of specific system subcomponents. To complicate matters, individual subcomponents may have several competing models whose query cost and accuracy payoff for system-wide prediction is opaque. For example, there may be numerous ways to identify macroscopic closure terms that are informed through analysis of a suite of microscale models.
We discuss how computational exploration-exploitation meta-algorithms from bandit learning can accomplish model selection and combination for computational budget allocation in scientific computing applications: An exploration phase is devoted to learning about model relationships and interactions, followed by an exploitation phase that uses information learned in exploration to make decisions about optimal model selection and subsequently provides full distributional information of stochastic outputs. This framework leads to flexible procedures, capable of managing disparate models and multi-modal data in adaptive and real-time scenarios. We also show how our exploration-exploitation approach can be used in complementary schemes for multilevel uncertainty quantification that exercise control variates and BLUE estimators. We will identify existing theoretical guarantees for such procedures along with promising and impactful directions for new analysis and algorithm development.
DMS Applied and Computational Mathematics Seminar
Apr 12, 2024 02:00 PM
ZOOM
Speaker: Yulong Lu (University of Minnesota, Twin Cities)
Title: Diffusion Models: Theory and Applications (in PDEs)
Abstract: Diffusion models, particularly score-based generative models (SGMs), have emerged as powerful tools in diverse machine learning applications, spanning from computer vision to modern language processing. In the first part of this talk, we delve into the generalization theory of SGMs, exploring their capacity for learning high-dimensional distributions. Our analysis shows that SGMs achieve a dimension-free generation error bound when applied to a class of sub-Gaussian distributions characterized by certain low-complexity structures. In the second part of the talk, we consider the application of diffusion models in solving partial differential equations (PDEs). Specifically, we present a physics-guided diffusion model for reconstructing high-fidelity solutions from their low-fidelity counterparts. This application showcases the adaptability of diffusion models and their potential to scientific computation.
DMS Applied and Computational Mathematics Seminar
Apr 05, 2024 02:00 PM
328 Parker Hall
Title: Deep filtering with adaptive learning rates
Abstract: Given the state of a system is not completely observable, filtering is concerned with state estimation based on partial observations of the system state. It enjoys many applications in the control of partially observed systems, target tracking, signal processing, statistics, and financial engineering. Devoted to the conditional distribution or density, the celebrated results of the Kushner equation and Duncan-Mortensen-Zakai equation produce nonparametric estimations of the conditional distribution/density. Approximating their solutions will suffer the curse of dimensionality. In this talk, we first introduce a filtering algorithm termed deep filtering based on the deep learning framework. Then we present our work on deep filtering with adaptive learning rates. Instead of approximating the conditional distribution or density, we focus on state estimation or conditional mean and convert the filtering problem to an optimization problem by finding the optimal weights of a deep neural network (DNN). This solves a long-standing (60-year-old) challenging problem in computational nonlinear filtering and has the potential to overcome the curse of dimensionality. We constructed a stochastic gradient-type procedure to approximate the weight parameters of DNN and developed another recursion to update the learning rate adaptively. We showed the convergence of the continuous time interpolated learning rate process using stochastic averaging and martingale methods and obtained an error bound for parameters of the neural network. Finally, we present two numerical examples to show the efficiency and robustness of our algorithm.
This is based on joint work with Prof. George Yin and Prof. Qing Zhang.